In the last couple of years, we have worked with several companies in the industrial sector to support their business operations with computer vision technologies. The customers have been from automotive, electronics, and process industries. In all cases, we have used computer vision to tackle demanding production and operations related challenges. In this blog, our aim is to describe the key learnings from these projects.
Computer Vision Technology – Utilization Speeding up
Computer vision (CV) has been used in many industries for decades. However it has been complicated to implement and it has required a lot of effort and specific expertise. In addition, creating true value with computer vision has been challenging.
Today we have easier access to data, more computing power and versatile AI tools, to build applications with reasonable cost for specific use cases. A better understanding of what can be achieved has encouraged customers to innovate new applications. Oftentimes advanced video analytics can provide a cost-effective way to automate simple monitoring tasks and free human labour for more productive activities.
At Top Data Science we continuously follow CV technology development. State-of-the-Art deep learning advancements including self-supervised pretraining, active learning, and model pruning have been successfully utilized in our solutions at production-level. As open source tools are improving and leading platform providers are also offering productized development environments, we align ourselves to create business value by selecting the most suitable technology stack for a given problem and building our solutions on top of that. We also have the ability to further develop the core algorithms together with our mother company Morpho Inc., which has been providing CV products to their customers since 2004.
Defining the Business Problem – Capturing the Objective
The best results in AI projects are reached when the business problems are clearly defined. Having a clear set of expected outputs helps to build algorithms for the specific problems. The following examples outline some actual problems in different business cases.
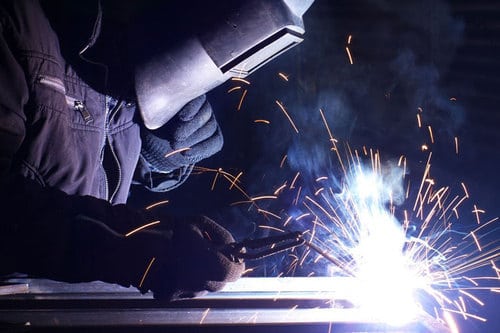
In the automotive industry, manufacturers need to conduct rigorous testing activities as part of their quality control processes. This testing is often a time and human resource consuming activity. In one of the recent customer cases, we focused on automating the analysis of welding seams, reducing the time spent on quality inspecting each part. The solution allows improvements in both productivity and quality. In providing easy to use tools for the daily routine tasks, we continue to emphasise the importance of supporting the actual workers to do their jobs better. The human aspect is very important to get people’s commitment and support when deploying AI in practice.
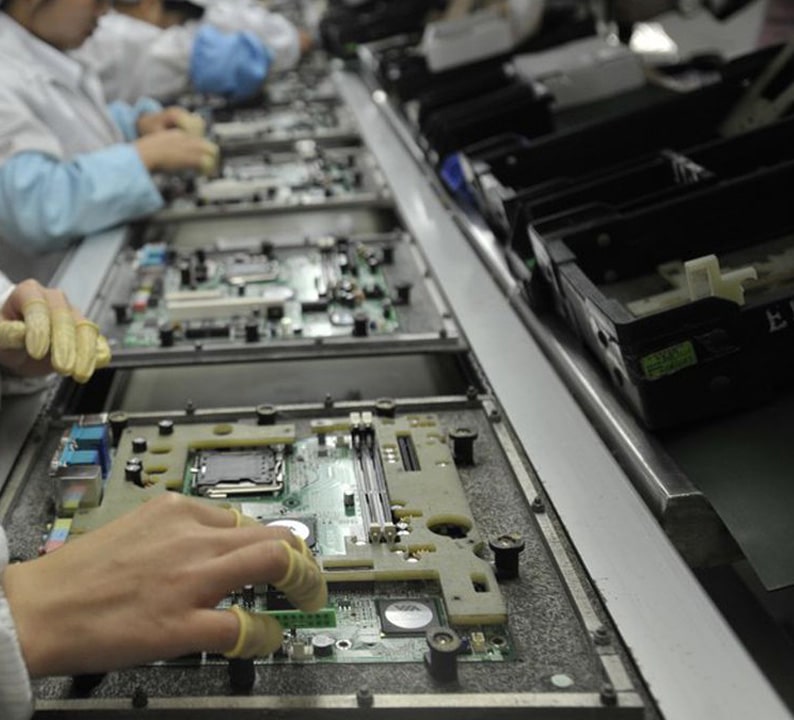
In electronics and the heavy machinery industry we need to account for complex multi-stage assembly processes. Computer vision can be used to monitor the progress of the work and notify users in real-time during the process, if something is not correct in the assembly, e.g. screws are missing or critical components are not in place. By providing such an application to our customer, they were able to save costs and improve throughput and assembly quality.
Choosing the Right Approach and Right Tools – Insight and Experience Needed
In the world of AI, it is crucial to pick up the right approach and tools to solve the defined problem. This is where the experience from several executed projects can be very helpful. The knowledge of different methodologies that could work in solving the particular problem and what is the right way to apply it is very beneficial in building the optimal solution.
Sometimes you also need to be ready to change the approach if the use case requires a totally new way of utilizing CV technologies. The data, the operative environment, IT infrastructure requirements (on-premise/cloud/edge), and potential integrations, all may require many iterations to find the optimal resolution for the business problem. Comparing different approaches is often the best way to find a workable solution.
Co-creation and working with subject matter experts is the key to finding the right approach. Data Scientists cannot be experts in industrial processes and typically process experts are not deeply involved in data science. Good communication, problem-solving skills, and common understanding about the problem are very crucial elements for success.
To Solve a Computer Vision Task You Need Appropriate Data – Multiple Approaches
All AI projects start with an appropriate task and required data definitions. The data is a key parameter affecting the performance of the algorithms. Most of the computer vision tasks require good quality and properly annotated data to get the algorithms to reach targeted accuracy. Still, in each case the requirement for data depends on the use case. Also, we should note that in order to get started even a limited amount of data can be used.
Once the data requirements have been defined, there are various ways to tackle the data challenge. These include utilizing publicly or commercially available datasets, annotating data by using annotation tools that support active learning, or acquiring annotation services from companies that specialize in such tasks.
Sometimes organizations do not have the experience to define data requirements or do not have the resources and time to invest in a good annotation. We have noticed that in some cases, annotations should be performed by subject matter experts in order to reach the desired quality. Top Data Science is able to provide expert help in analyzing given tasks and defining data requirements. Furthermore, there are ways to automate some of these processes. For example, we have designed and implemented a solution that uses existing CAD models to generate photo realistic images that can be utilized for training machine learning models.
Tackling the scaling up challenge – key to achieve ROI
Most of the AI projects still end up stopping at the Proof-of-Concept stage. Why is this the case? According to our experience, the reasons could be the following:
- Lack of clear understanding of the potential value of AI to the business
- Lack of competent resources – how to buy AI or establish own competence pool
- Lack of support from the management to invest in developing AI capabilities
- Uncertainty related to the matureness of AI in specific application area
In recent research conducted by Athina Kanioura and Fernando Lucini (https://hbr.org/2020/04/a-radical-solution-to-scale-ai-technology), they mention three key success factors in the path of scaling AI technology:
- Pivot to piloting — A piloted technology takes a fully-baked capability and launches it directly into the real world (albeit on a smaller scale).
- Commit to Action – Organizations should consider just a few valuable projects and focus on doing the proper research and putting them into production.
- Make sure that the right team is in place
At Top Data Science our vision is to enable a more autonomous future to our customers through State-of-the-Art, scalable AI solutions. Also, as an active co-creation partner for our customers, we have seen the transition from Proof-of-Concepts and pilots to applying scalable AI as an active part of the everyday life at the customers operations.
We are happy to share our knowledge and expertise to get you started.
Writers are:
Vesa Jaakkola, Sales Director, Top Data Science
Oguzhan Gencoglu, former Head of AI, Top Data Science